Advancing Atmospheric Modeling with AI-Driven Aerosol Optical Properties Calculations
Aerosols—tiny particles suspended in the atmosphere—have a significant impact on weather climate by scattering and absorbing solar radiation. Accurate modeling of aerosol optical properties (extinction coefficient, single scattering albedo and Asymmetry parameter) is essential not only for climate predictions but also for applications in weather forecasting and energy systems. Traditional methods based on Mie theory, while precise, are computationally expensive, and simplified look-up tables often sacrifice accuracy. Developed by researchers from ART group at IMKTRO, MieAI, a machine learning-based optics model, offers a faster and more efficient alternative for calculating these properties online in atmospheric models like ICON-ART.
MieAI uses a neural network to model how aerosols of various sizes and compositions interact with radiation. It processes input data such as aerosol size distribution and refractive index through multiple layers in the neural network to predict optical properties like scattering and absorption coefficients. Initial testing shows that MieAI’s predictions closely match those from traditional Mie theory as shown in Figure 1 below and is faster upto 3 orders of magnitude, making it a promising tool for atmospheric simulations.
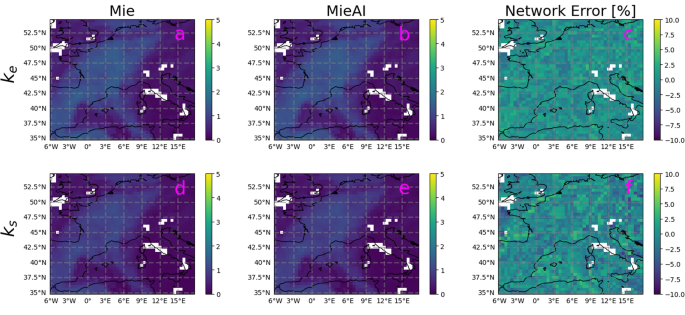
MieAI has already been integrated into ICON-ART model.
However, it is undergoing extensive testing and validation before its public release as a component of ICON-ART modeling system. Once fully validated, it will help scientists simulate real-world events like volcanic eruptions, dust storms, and wildfire smoke more efficiently. This will provide a better understanding of aerosol effects on climate and weather patterns, ultimately supporting more accurate climate predictions and better-informed decision making.
References
Kumar, P., Vogel, H., Bruckert, J., Muth, L. J., & Hoshyaripour, G. A. (2024). MieAI: a neural network for calculating optical properties of internally mixed aerosol in atmospheric models. npj Climate and Atmospheric Science, 7(1), 110, doi: 10.1038/s41612-024-00652-y