New mathematical methods to decipher African meteorology
Africa is the largest continent in the tropics and home to over a billion people, many of which depend on rainfed agriculture. Therefore better weather predictions, particularly of precipitation, as well as more reliable assessments of climate change are key to better planning in the short and long term. Unfortunately, many past studies have demonstrated substantial difficulties of state-of-the-art computer models to represent the complex meteorology of tropical Africa, which is characterized more than anywhere else by potent, long-lived and intense complexes of thunderstorms stretching for hundreds of kilometres. Increasing the spatial resolution of these models, which increases their computational costs enormously, leads to measurable improvements but does not appear to be the ultimate cure of the problem. “It is almost embarrassing for our discipline”, says Peter Knippertz, leader of the “Atmospheric Dynamics” group at IMKTRO, “that we are still not able to provide forecasts that are good enough for day-to-day decision-making, to a large portion of the African population.”
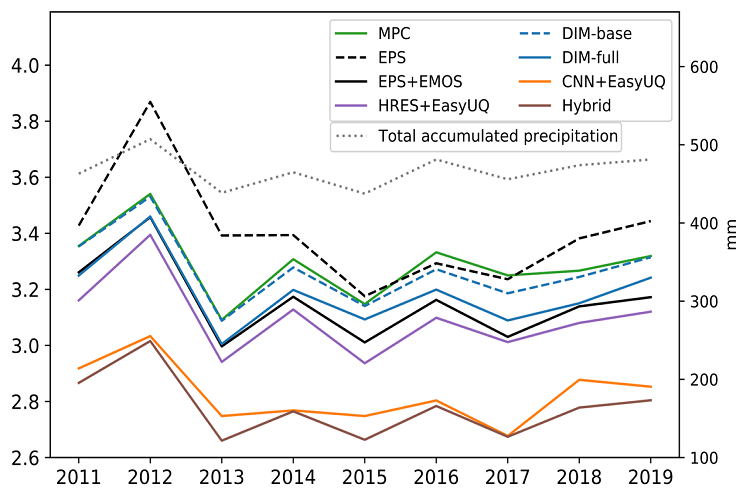
To make progress, the group has engaged increasingly in recent years with colleagues from mathematics and related disciplines to explore new approaches to this old challenge. One such approach is a so called “statistical emulator”. The idea behind this is that weather and climate models contain many uncertain parameters like for example the mixing efficiency between thunderstorm and ambient air. “Testing all plausible parameter combinations with the numerical computer model is too costly," says Matthias Fischer, a PhD student in the Dynamics group working on an emulator for Africa. "Instead, we systematically select specific parameter combinations to run the numerical model. We then create a statistical surrogate to make it computationally efficient to display all dependencies and sensitivities.” A publication about this work has just appeared in Weather and Climate Dynamics (Fischer et al. 2024). A follow-on study that tries to estimate an optimal parameter set by comparing the emulator output to observations will be submitted soon.
Another new approach is to replace the conventional physics-based weather prediction models through algorithms based on classical statistical methods and machine learning. A first successful attempt for probabilistic forecasts of precipitation occurrence based on simple logistic regression and using rainfall fields of previous days as input was already published in 2021 (Vogel et al. 2021), followed by a substantial refinement in 2023 (Rasheeda Satheesh et al. 2023). Currently, we are working on more sophisticated machine learning approaches that take into account additional predictors such as large-scale atmospheric waves filtered from rainfall data (Rasheeda Satheesh et al., to be submitted soon) or additional atmospheric fields such as vertically integrated moisture and convective instability (Walz et al. 2024; see figure). “The success of these new and relatively cheap machine learning models is phenomenal”, says Knippertz, “and we are working to make such approaches available to African forecasters soon.”
References
Fischer, M.; Knippertz, P.; van der Linden, R.; Lemburg, A.; Pante, G.; Proppe, C.; Marsham, J. H., 2024: Quantifying uncertainty in simulations of the West African monsoon with the use of surrogate models. Wea. Climate Dyn., 5, 511–536, doi:10.5194/wcd-5-511-2024.
Rasheeda Satheesh, A.; Knippertz, P.; Fink, A. H.; Walz, E.-M.; Gneiting, T., 2023: Sources of predictability of synoptic-scale rainfall during the West African summer monsoon. Quart. J. Roy. Meteorol. Soc., 149(757), 3721–3737, doi:10.1002/qj.4581 .
Vogel, P.; Knippertz, P.; Gneiting, T.; Fink, A. H.; Klar, M.; Schlueter, A., 2021: Statistical forecasts for the occurrence of precipitation outperform global models over northern Tropical Africa. Geophys. Res. Lett., 47, e2020GL091022, doi:10.1029/2020GL091022.
Walz, E.-M.; Knippertz, P.; Fink, A. H.; Köhler, G.; Gneiting, T., 2024: Physics-based vs. data-driven 24-hour probabilistic forecasts of precipitation for northern tropical Africa. Mon. Wea. Rev., in press, doi:10.1175/MWR-D-24-0005.1.